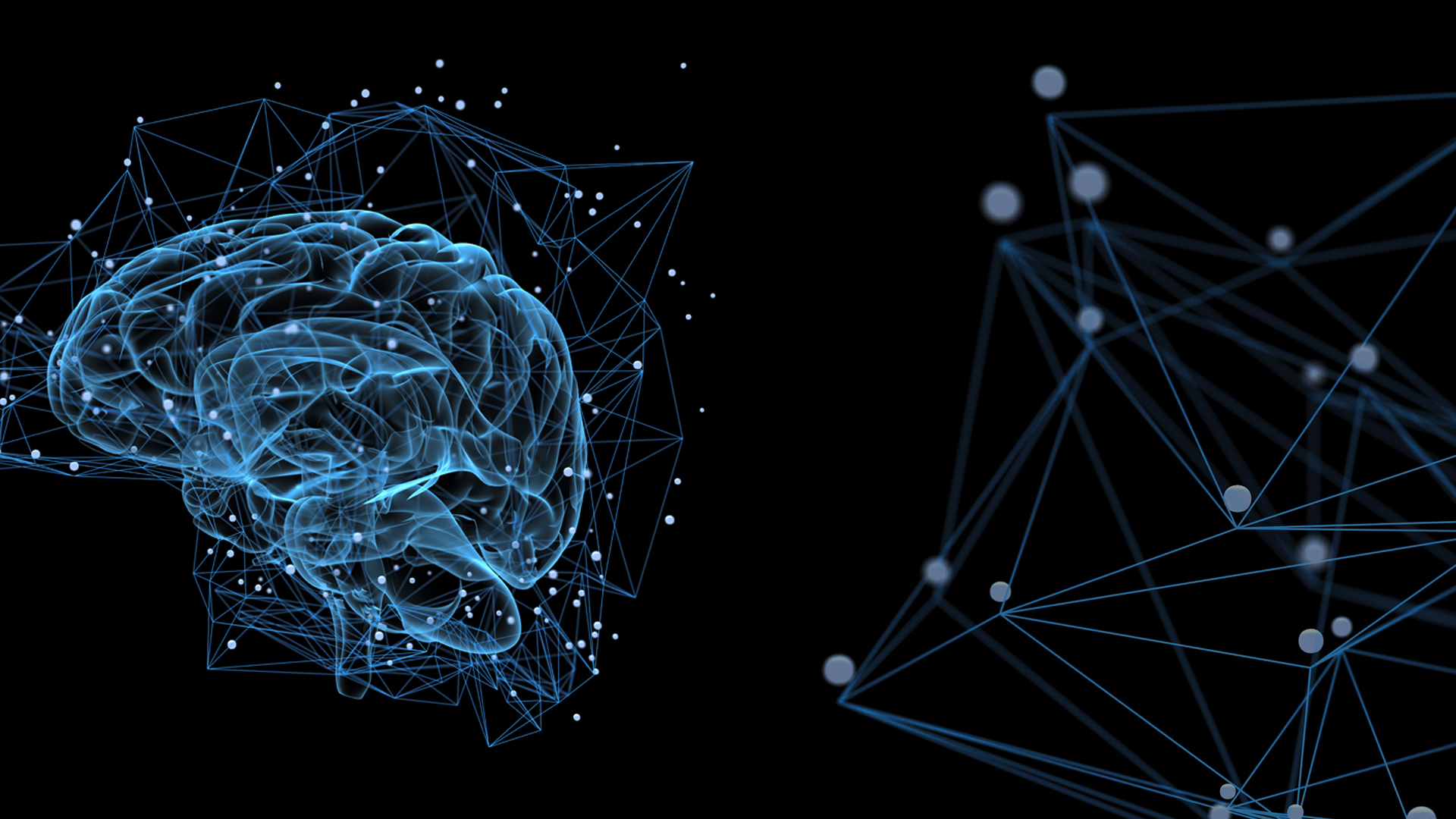
Modern machines and production facilities supply vast amounts of data. The art lies in generating added value from this information. With artificial intelligence, correlations can be formed and processes can be optimized by self-learning. To do this, however, mechanical engineers and plant operators need a future-proof technological basis on which they can introduce their AI competence step-by-step and in a scalable manner. A powerful and open control platform is the basis for the implementation of artificial intelligence.
A current study commissioned by the Federal Ministry of Economics and Energy (BMWi) puts the additional gross value added of the manufacturing industry influenced by artificial intelligence at around 32 billion euros over the next five years. This corresponds to one third of the total growth forecast for this sector over this period. According to the study, around 25 percent of large companies, but only 15 percent of small and medium-sized enterprises use AI technologies in the manufacturing sector.
As a basis for the use of artificial intelligence, data from machines such as temperature, vibration, throughput, energy consumption and other sources such as images of quality processes must first be collected over as long a period as possible. In modern production plants, the technological prerequisites are now largely met by the large number of sensors and the networking of machines via Industrial Ethernet. The data volumes generated are so large that they can hardly be evaluated, correlated and interpreted with human power. However, artificial intelligence can be used to bring all the data together and then create the much-cited added value. These can be new data-based services as well as knowledge management, product improvements or optimized utilization of machines and production lines. And the latter starts with the detection of emerging problems and extends to the independent adaptation of the control system to flexible, changing and modular production scenarios.
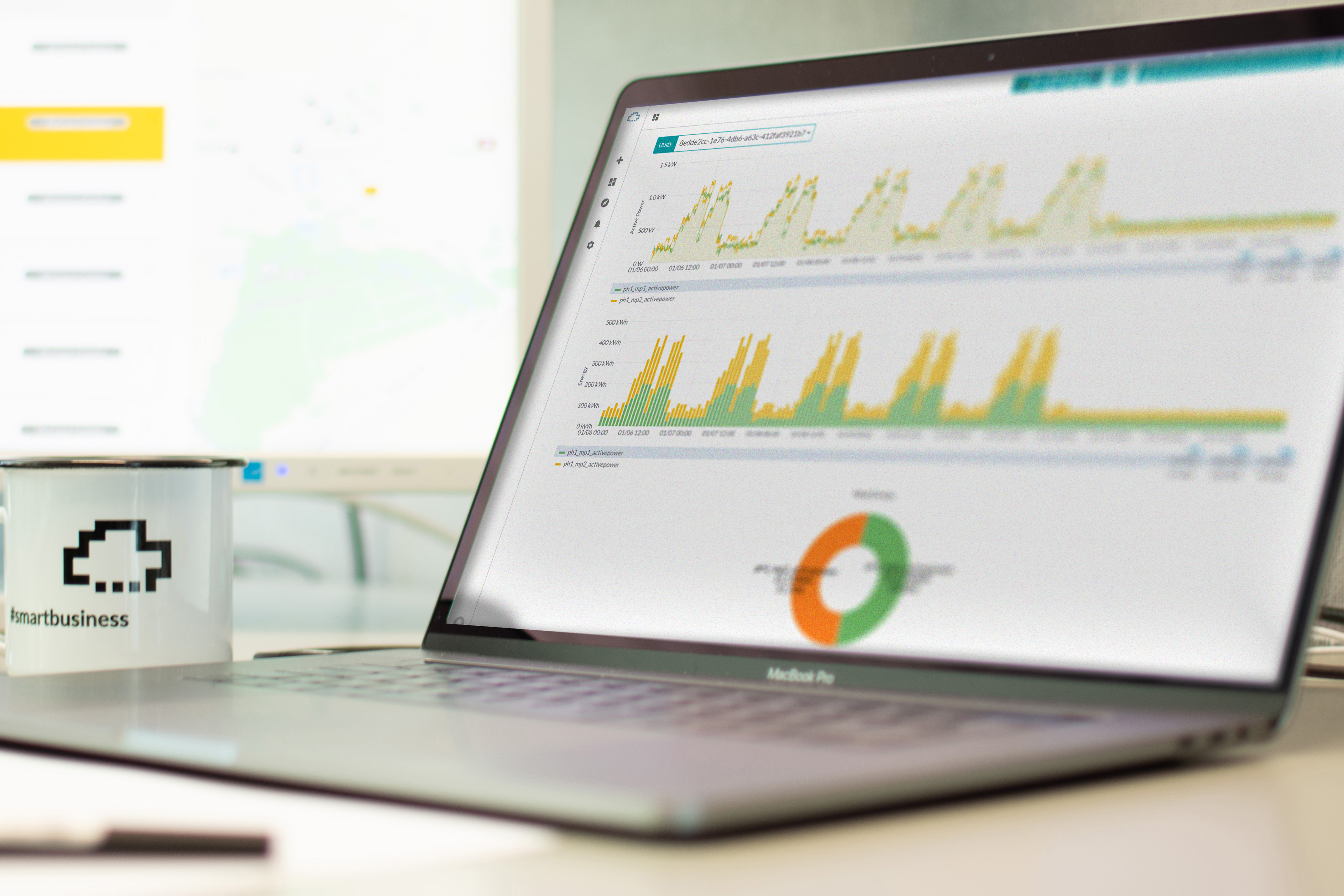
Artificial Intelligence, Machine Learning and Deep Learning ?
With artificial intelligence, it is currently the same as a few years ago with the term Industry 4.0: everyone is talking about it, but very few people know what it’s all about. In connection with AI, there is also a lot of talk about Machine Learning and Deep Learning technologies.
Artificial intelligence (AI) is the general term used in computer science and neuroscience, which also includes the technologies machine learning and deep learning. Artificial intelligence is the ability to think like a human being and imitate intelligent behavior – this includes characteristics such as self-learning, self-reflection and empathy. A machine with artificial intelligence therefore makes decisions independently. A famous example of AI is the 1997 victory of IBM’s Deep Blue in chess against Gary Kasparov. In 2011, IBM’s Watson computer achieved a symbolic victory in the US game show classic Jeopardy against the best players. Another milestone is the victory of Google’s DeepMind in the Japanese board game Go against the champion Lee Sedol.
When it comes to Machine Learning (ML), then it is a concretization of artificial intelligence. Especially in the industrial environment, ML is regarded as the driving factor for developments in the field of AI. ML learns to determine solutions to problems on the basis of data. The more data an ML system has at its disposal, the better it can optimize its algorithms – the accuracy increases to a certain degree over time. In some cases, the learning phase is also based on human decisions. The learning phase enables the system to recognize comparable patterns and derive the optimal action from them. ML is based on a variety of mathematical methods of pattern recognition. An example of ML is the recognition of defects in images during quality control in production.
Deep Learning (DL) can in turn be described as an advanced subcategory of machine learning. This technology relies on artificial neural networks. Whereas ML is based on mathematical logic and the decomposition of data flows into hierarchical structures, DL uses nature with biological neural networks as a model. Here, data is processed in a similar way to the neurons in the human brain – this is done via several neural layers, each of which handles different problems. DL technology is used when ML reaches its limits. While ML algorithms have a limited learning capacity, regardless of the amount of data available, DL is constantly improving its performance with more data. For example, DL enables robots to handle objects that are irregularly shaped or arranged. Autonomous driving is also an example of DL.
Where does AI make sense in production processes?
AI is now used in a wide range of applications in production processes with high economic benefits. These include predictive maintenance of machines and systems, collaborative and context-sensitive robotics, as well as production and capacity planning and quality control. According to the BMWi study and the management consultancy McKinsey, four central core areas have been identified in which AI will be used beyond the usual extent within production:
- AI-based Predictive Maintenance Systems: Predictive maintenance gets better prediction and prevention of machine failures through the combination of data from sensors, maintenance logs and external data sources.
- Collaborative and context-sensitive robotics: Production throughput is significantly improved by AI-optimized robotics.
- Increased efficiency and earnings: The linking and evaluation of numerous Data across machine groups, production sites and ERP levels allow cost-optimized production through AI.
- AI-based quality control: More efficient and reliable data processing through machine learning ensures continuous improvement of product quality and reduces reject rates and testing costs.
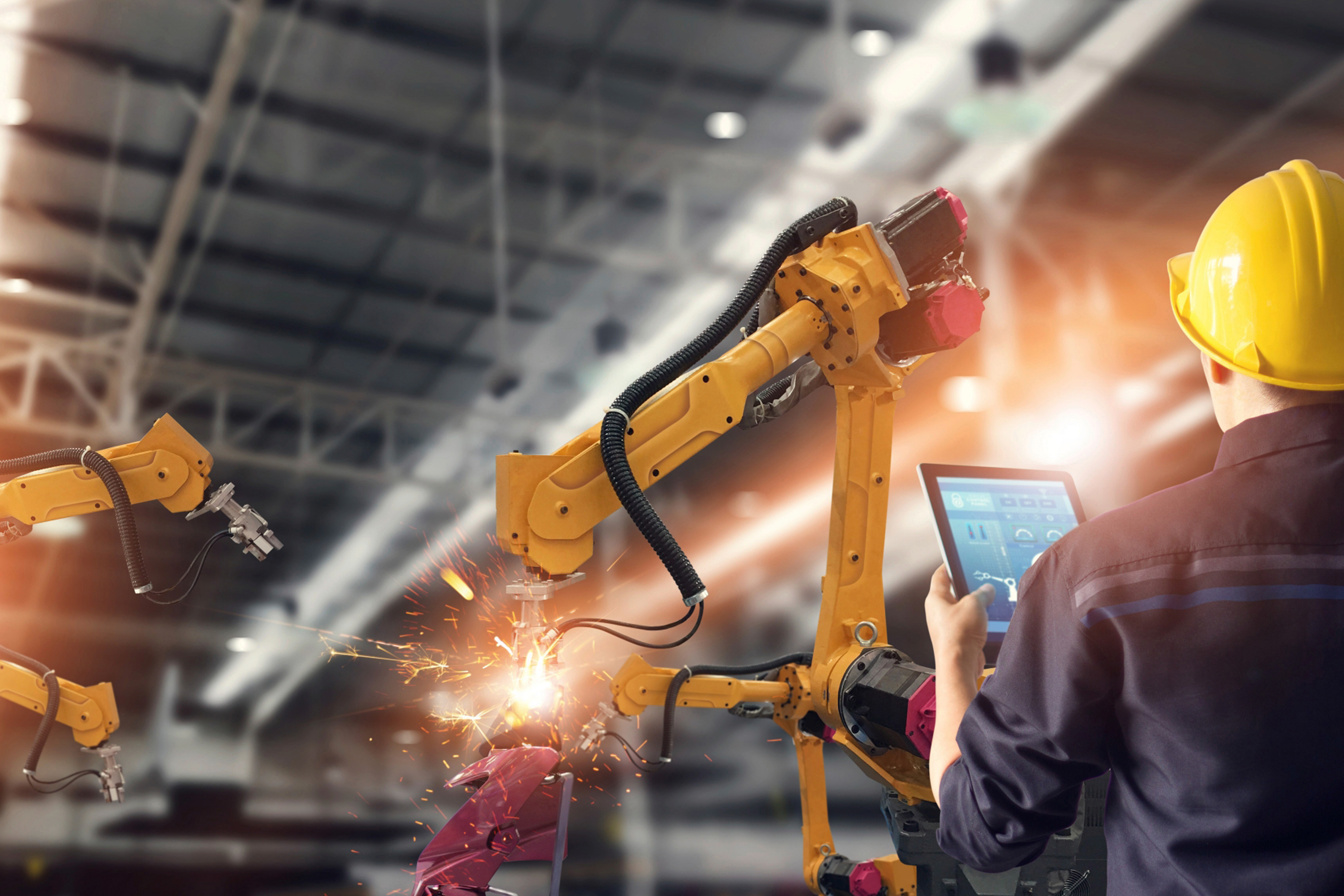
“Machines and robots cannot possibly be extensively converted and reprogrammed for each individual personalized product. They must be able to adapt to changed conditions independently using learning algorithms”. Dr. Werner Kraus, Deputy Head of Robot and Assistance Systems at Fraunhofer IPA
With AI, quality problems can be detected before the product is even produced. This can be done, for example, by image evaluation of the supplied raw materials via machine learning, as well as by the imminent exceeding of machine tool tolerances during the next night shift.
Artificial intelligence is also suitable for the transfer of knowledge from experienced and older employees to new and younger colleagues. The use of modern technology should be promoted in this area, particularly in view of the shortage of skilled workers in almost all areas: When it comes to setting up, operating, maintaining or repairing machines and systems, specialists document the processes and important steps by recording them with cameras. Untrained employees then receive support via augmented reality. Using image recognition via AI, the untrained or new employees are then shown the appropriate instructions for action via data glasses and augmented reality. Artificial intelligence also supports employees in production through speech recognition. For example, they can use data glasses to display operating and assembly instructions directly on the machine using voice commands.
“AI systems can provide context-sensitive information, analyze motion sequences, verify the result of certain motion sequences and relieve humans of certain inputs during the production process. We therefore see great potential in AI-based applications, particularly in the area of knowledge management,” adds Prof. Dr. Martin Ruskowski, head of the research area Innovative Factory Systems at DFKI and an expert on AI in production. AI also reduces the programming and engineering effort for control and automation solutions. Particularly in the case of modular and changing production conditions, control systems with AI algorithms can adapt more easily and self-learning.