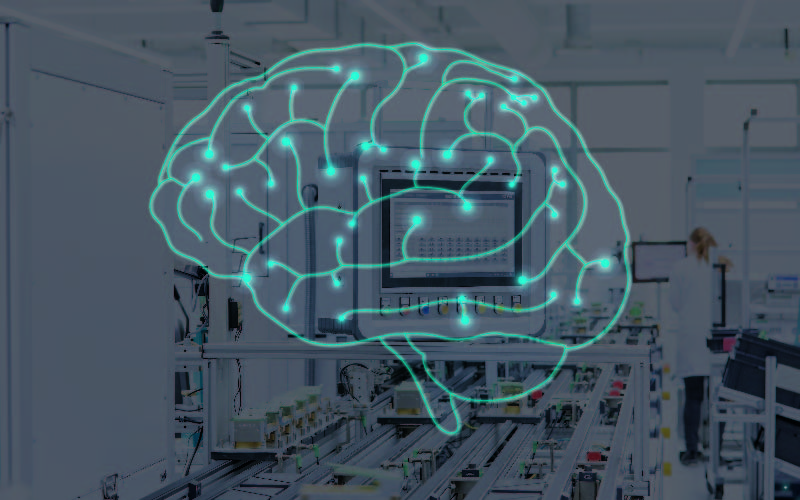
The potential for optimizing processes and developing new products and services with the help of artificial intelligence (AI) has long been recognized.
It is no longer the preserve of big tech and digital giants but is increasingly being used in many different industries. The 2020 MIT study “The global AI agenda” shows that the willingness to use artificial intelligence in business is steadily growing among most companies. For many entrepreneurs, AI is not a ´nice to have´ but rather the basis for entrepreneurial action.
“For us, AI is a foundational technology that in the next couple of years will be found in the vast majority of our propositions.” (Jeroen Tas – Chief Innovation and Strategy Officer at Philips)
Smart and sustainable analysis of raw data generated by many sensors is becoming a core function of professional IoT applications. It enables machine learning by developing smart solutions to problems and building intelligent systems. In this way, quick decisions can be made, especially in critical situations. This helps to implement solution-oriented measures.
How it works
The core component of industrial automation systems is a classic PLC (Programmable Logic Controller). It processes the inputs of the automation system and generates outputs to control the processes according to the programming. And all this with the requirement of real time!
This requirement can be negatively influenced by additional tasks – for example, complicated calculations in the environment of artificial intelligence (AI) or machine learning (ML) – due to an excessive load on the PLC. One solution to this problem is, to shift computationally intensive tasks to external add-on modules that relieve the PLC.
This requirement can be negatively influenced by additional tasks – for example, complicated calculations in the environment of artificial intelligence (AI) or The results, calculated by the add-on module, are then returned to the PLC program for further processing. In the same way, the variables required by the PLC are to be transferred to the external computing unit as input values of the AI. To realize this process, the control application running on the PLC must be able to communicate with the external computing unit.
Side Fact | Artificial Intelligence = Branch of computer science that deals with the automation of intelligent behavior and machine learning.
Side Fact | Machine Learning = Subfield of artificial intelligence that generates artificial knowledge by recognizing patterns and regularities and deriving suitable solutions from them. This knowledge learns from previous experience and thus continues to develop independently.
Preferred Programming Language combined in one Tool
Both disciplines, programming, and automation, work closely together in the development of AI-models. The programmer uses his preferred language Python for his work. However, this differs considerably from the IEC61131 languages known in the automation world, such as structured text, flowchart, ladder diagram, function block language or instruction list. For successful collaboration of both disciplines, it is essential that each participant can work on the AI-model in his or her preferred language.
It is therefore worth investing in a controller with an open ecosystem. This supports both parties in their joint work on artificial intelligence.
Our tip: We link our latest blog post about “open ecosystems” at the end of this blog post – enjoy reading!
Learn, Train, Perform
When applying machine learning methods, a distinction must be made between learning, training, and running a finished AI-model.
The effort and computational power required to create an AI-model are significantly higher than the computational power required to use the finished model. In addition to programming the algorithms, the preliminary work required to create complex AI projects also includes the collection and labeling of data. This often requires a great deal of data, which must be classified accordingly.
In the preparation phase, the data is divided into training and test data. During the development process, the training data is used to optimize the AI-model. The test data is used to check which results the created model delivers with the unknown data and to what extent they need to be adjusted.
The data scientist selects the appropriate machine learning method from the many AI options, for example, a decision tree or a deep neural network (deep learning). He then uses the data to develop a suitable program.
Side Fact | Deep Learning = Advanced form of machine learning. With the help of analyzed data and a neural network, the machine can repeatedly link what it has learned with new content. Further it can keep learning new things, making predictions and decisions.
On-Site Processing of large Amounts of Data
One example of successful deep learning is intelligent image recognition. It offers a solution for optimizing road traffic. Especially in inner cities (#smart cities). To create an image of the traffic situation, data – for example, images from traffic cameras – must be recorded by suitable sensors and then directly processed and evaluated in the next step.
If an edge computer system is used for this process, it has the advantage that large volumes of data – such as videos – do not have to be forwarded to the cloud via an Internet connection but can be processed directly on site.
Artificial intelligence also offers a decisive advantage in predictive maintenance. Here, the PLC collects data determined by vibration, temperature, or acoustic sensors, for example, and transmits it to an AI extension module via the controller’s interfaces. The AI-module uses the information from the sensors as input data for the corresponding artificial intelligence model, for example, the neural network or regression. With the results of the AI, the PLC can now bring the machine into a safe state in the event of an imminent danger or send a message to maintenance personnel to check the machine in good time.
Top Three Benefits of Artificial Intelligence in Manufacturing
Faster decision making: Operational decisions are primarily data driven. They must be made in real time and have a major impact on a company’s profitability. Thanks to IoT sensors, data can be collected and analyzed in large volumes, and the real-time requirement applies. This helps to make the right decisions at the right time, saving costs and time.
Safety: One of the highest-risk work environments is manufacturing. Thanks to machine learning, AI can make particularly dangerous jobs more predictable and safer. This helps protect people and prevent unintended accidents.
Cost Reduction: Using AI technologies and the resulting improvement in companies’ analytics capabilities can make more efficient use of resources. Similarly, predictive maintenance helps provide more accurate forecasts to save effort and money.
To sum it up:
In times like these, businesses are facing more challenges than ever (e.g., lack of product supply, downtime, skills shortages). Thanks to artificial intelligence and machine learning, companies can not only increase their efficiency and adapt to changing market situations, but also create necessary stability.
This is necessary to be able to withstand the current challenges on the market and thus consolidate the company’s position in the competitive environment.
You want to read more about open ecosystems and they reasons, why they chance the way we work together? Then we invite you to read our latest blog post about open ecosystems >>